Principled Data Governance for Today’s Business Environments
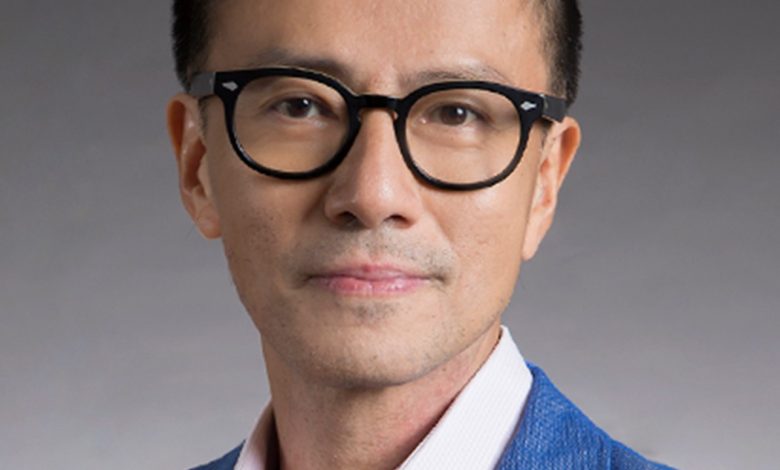
By: David Chan, Managing Director, Adnovum Singapore
A data governance framework is essential to ensure that an organization’s data assets are accessible when needed, up-to-date, and secured in an environment in accordance with an organization’s internal data standards and regulations.
However, the reality of everyday business activities and complexities mutes this ideal. No single organization interfaces and applies data in exactly the same way. Research has revealed that close to 22% of software decision makers in the Asia Pacific (APAC) region, said the lack of alignment between IT and business outcomes was one of the biggest challenges in executing software strategy.
Moreover, organizations today pool more volumes of data, data assets, and confront more complexity in data security and compliance. The roadblock is clear: in today’s data-driven business environment, there is no one-size-fits-all data governance program.
While APAC sets the tone to overall data management and governance — more centralized than its global counterparts, data quality and usable data remains a challenge. As cross-functional business operations continue, inconsistent data taxonomies become an impediment, affecting data quality and data useability.
Today, there are time-tested data governance principles that leadership teams can apply to build data governance programs.
Contextualize your data
What are the goals of the business unit or team? What direction is this organization heading towards in terms of the general business? These big-picture organization questions play a fundamental role in determining the context to apply data-driven work in everyday activities. Data governance challenges are dependent upon the context it is operating within.
To determine which sources of data to incorporate into work, it is helpful to have a clear definition of what is the desired business outcome. Is this data supposed to impact top-line revenue, bottom-line profitability, or possibly both? The desired business outcome will drive the fundamental decisions being made on which data to collect, and why this data is being collected.
Answering these questions will bring clarity by materializing data challenges into the realms of everyday concerns.
Establish your data governance framework
Today, the momentum behind data-driven operations has led to numerous side effects. These include data sprawl, inadequate data quality during analysis transformation, and cases of circumventing compliance regulations locally and internationally. In a survey by Gartner, 55% of decision makers found the lack of a standardized approach to data governance as the key challenge in transforming data use cases into clear business value. The paradox is clear: generating and collecting more data alone does not create the forward momentum for insights and business value to be unlocked.
Each organization identifies and applies data in its own unique way to derive the best business value it deems profitable. Transparency and accountability are therefore the bedrocks in defining and designing a data governance program.
The framework must address fundamental questions: Who owns each data asset? What roles and responsibilities must the data asset owner fulfil? How do we ensure data is collected, curated, and qualified for use across the business?
While these questions may seem elementary, we are currently experiencing the ramifications of these questions today at an accelerated pace. To contextualize this concern into our present moment, more aspects of professional work today are already embedding the use of generative AI to transform workflows.
When data is correctly collected, curated, and formatted, it can be used for analytics and as training datasets for machine-learning algorithms in predictive analytics.
These generated insights play crucial roles in decision-making for financial market strategies, business strategies, and even the recommendation engines that power our online shopping or entertainment experiences. These are massive positive benefits to the broader economy.
Follow the data trail from governance to protection
A comprehensive data governance program also ensures data integrity across touchpoints and parties interacting with the data.
Establishing common data quality metrics solidifies data integrity, a key component of your organization’s data governance program.
Are there mechanisms in place to intervene when error rates in datasets exceed acceptable levels? What are the quality levels in data definition, metadata and data catalogues? What are the possible quantitative measures to define data completeness and data consistency internally as an organization?
Likewise, an effective data governance program adapts and complies with local and international rules and regulations. Safeguarding data and guaranteeing that it is used in line with all applicable external standards cannot be overstated.
For instance, in Singapore, organizations are obligated to comply with the Data Protection Obligations under the Personal Data Protection Act (PDPA) to protect data entrusted to them by customers, clients, and employees. They can demonstrate a greater degree of compliance with the PDPA by following through with the Data Protection Trustmark (DPTM). These accountability tools demonstrate responsible data usage to clients, business partners, and regulatory institutions.
As more of everyday business activities and professional work involve integrating tools, external data assets, and connecting multiple stakeholders and users, data governance may tend to be placed as a lower priority. However, data breaches and inappropriate business usage of data assets may result in more lasting consequences than poor decisions and wasted resources.
A quality data governance framework is built upon an organization’s specific context in relation to how it serves other businesses and broader industry to create value. Without frameworks, there is a tendency to be overly protective of data, or isolate data within the organization. The possible use cases to explore across the value chain are reduced out of fear rather than trust. The true arbitraging power of data comes from implementing use cases that cut across different business units or organizations that impact entire value chains.